Operation of snowflake proxies with the standalone version in numbers
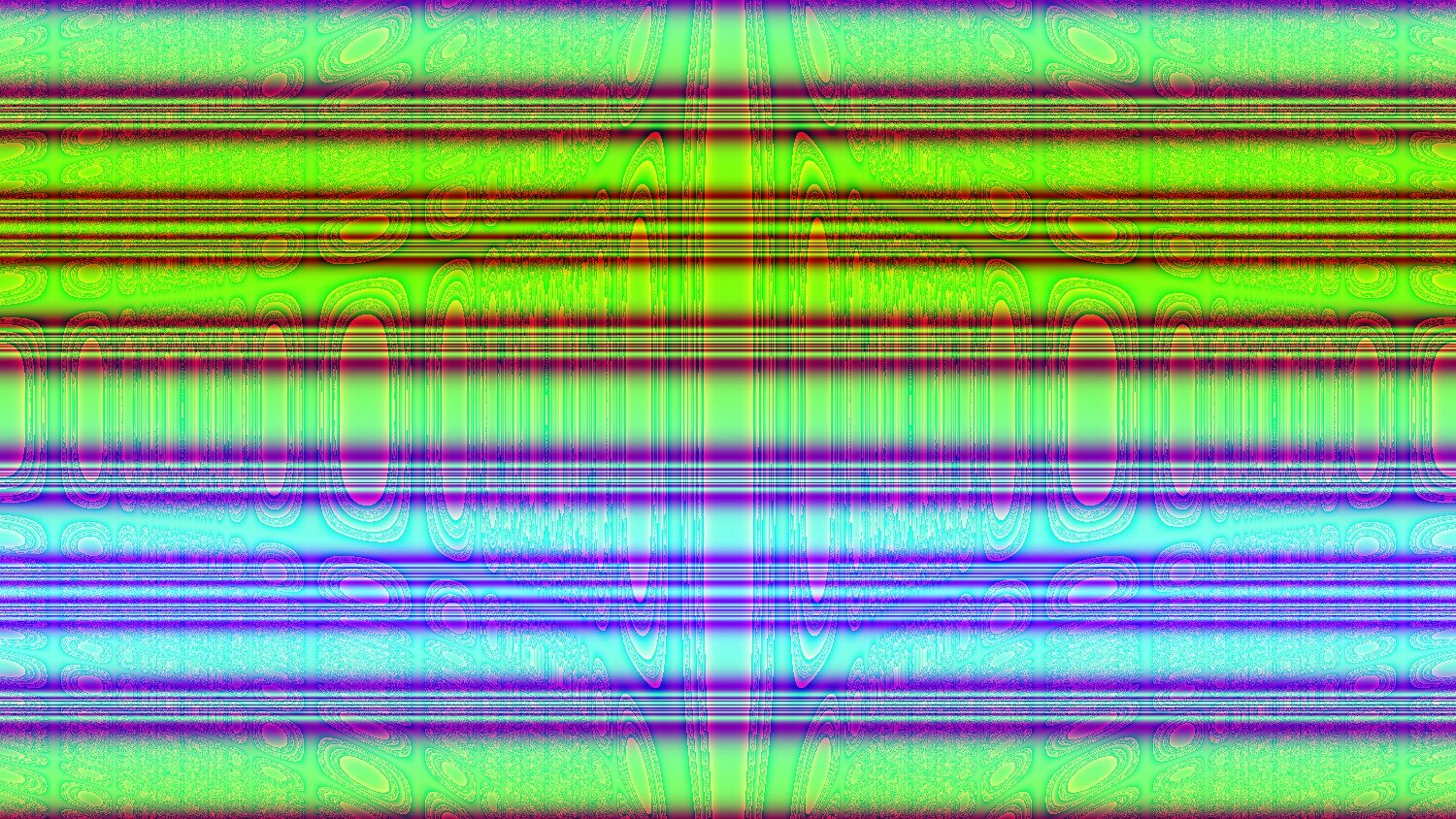
Snowflake bridges or proxies allow you to connect to the Tor network when it is blocked. This type of bridge adds to the alternatives and anti-censorship efforts of Pluggable Transports (PTs) such as obfs4 and meek-azure.
Snowflake proxies are operated by volunteers around the world, as with the other types of Tor network nodes.
There are three methods by which you can participate in the operation of snowflake proxies: in-browser extension, in a browser where WebRTC is enabled and via the standalone version.
The standalone version allows the installation of servers that function as snowflake proxies.
Statistical data on hardware resource usage and bandwidth consumption for four snowflake proxies are reported below.
Hardware and software features
For the operation of the snowflake proxies, 4 virtual machines with Xen paravirtualization and Debian 10 operating system were installed. Deployment was performed with the snowflake ansible role.
Nombre | Hardware |
snow1 | Memoria ram: 3072 MiB Procesador: 2 núcleos Disco duro: 10 GiB |
snow2 | Memoria ram: 1024 MiB Procesador: 1 núcleo Disco duro: 10 GiB |
snow3 | Memoria ram: 512 MiB Nucleo: 1 núcleo Disco duro: 10 GiB |
snow4 | Memoria ram: 512 MiB Nucleo: 1 núcleo Disco duro: 10 GiB |
Bandwidth results
As a result of the calculation and registration with vnstat, we had the following results for the months of May and June.
Nombre | Mensual | Total | Velocidad promedio |
snow1 | Mayo – 23.61 GiB Junio – 13.92 GiB | 48.84 GiB | Mayo – 75.73 kbit/s Junio – 72.90 kbit/s |
snow2 | Mayo – 22.19 GiB Junio – 10.51 GiB | 36.73 GiB | Mayo – 71.15 kbit/s Junio – 55.06 kbit/s |
snow3 | Mayo – 20.49 GiB Junio – 6.48 GiB | 31.21 GiB | Mayo – 65.71 kbit/s Junio – 33.96 kbit/s |
snow4 | Mayo – 15.52 GiB Junio – 8.44 GiB | 28.00 GiB | Mayo – 49.76 kbit/s Junio – 44.21 kbit/s |
As can be identified in the hardware characteristics there are differences in terms of processing cores and ram memory. Since the intention was to study the performance and performance according to different scenarios. In order to document which hardware can be more useful for the operation of the proxies and characterize the operation of the software on its performance and performance.
To obtain clues about the performance of the snowflake software and its relationship to the 4 scenarios, snow 1, 2, 3 and 4, we incorporated the details of CPU usage and system load during 12 hours of continuous operation into the analysis.
System load
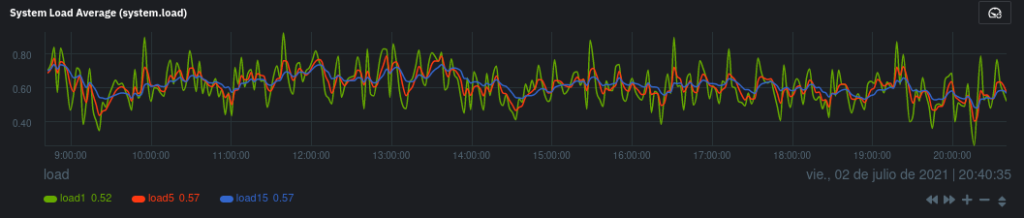
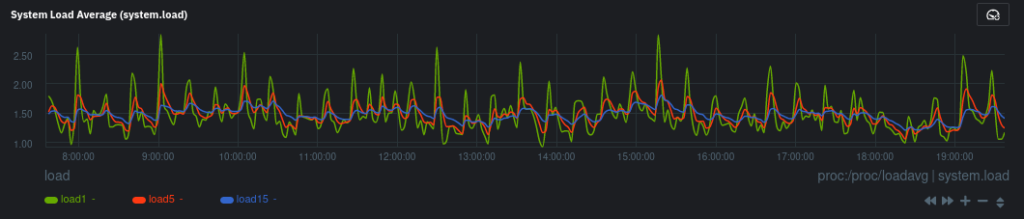
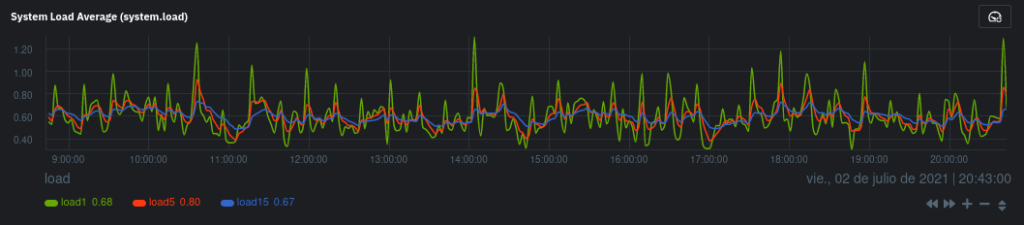
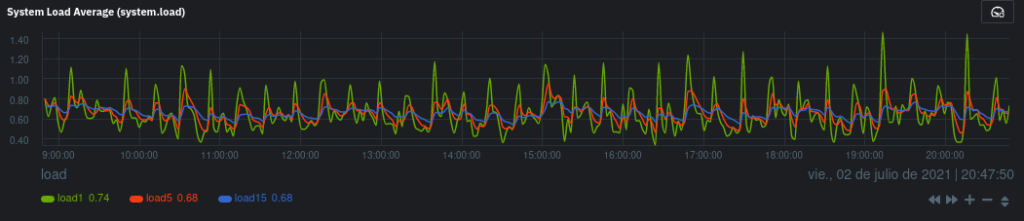
CPU Usage
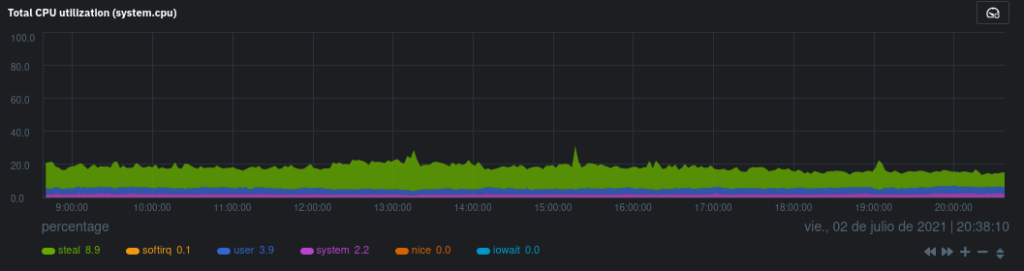
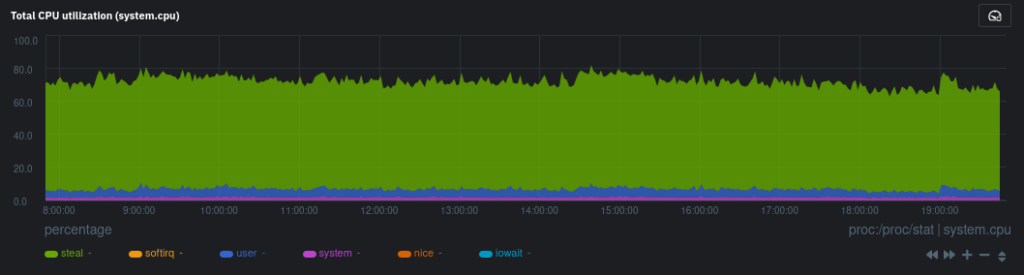
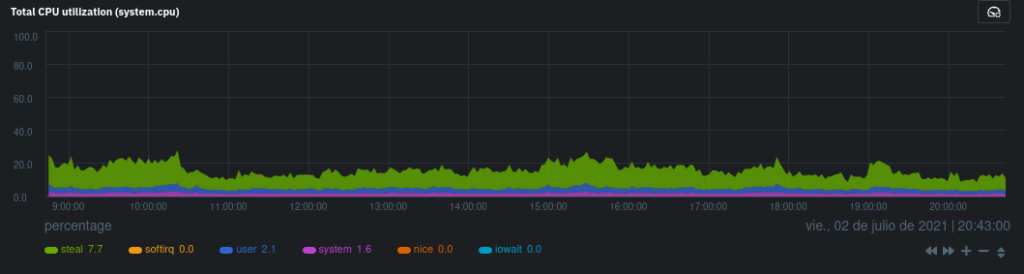
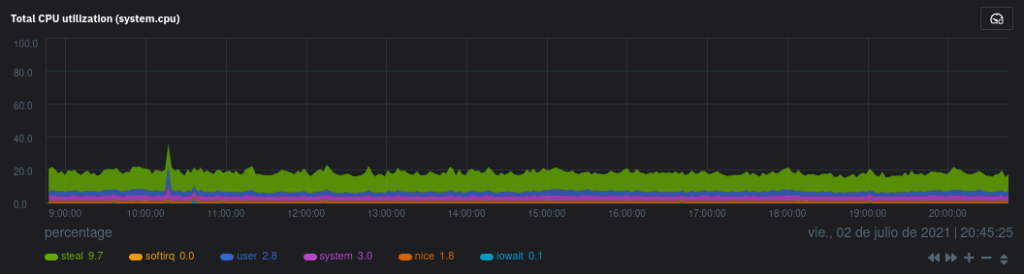
Memory ram usage
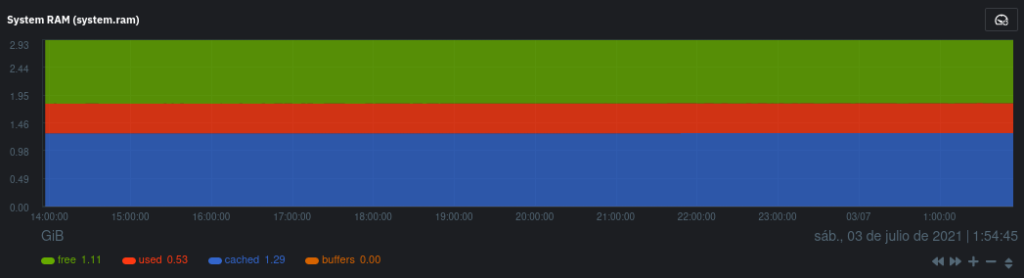
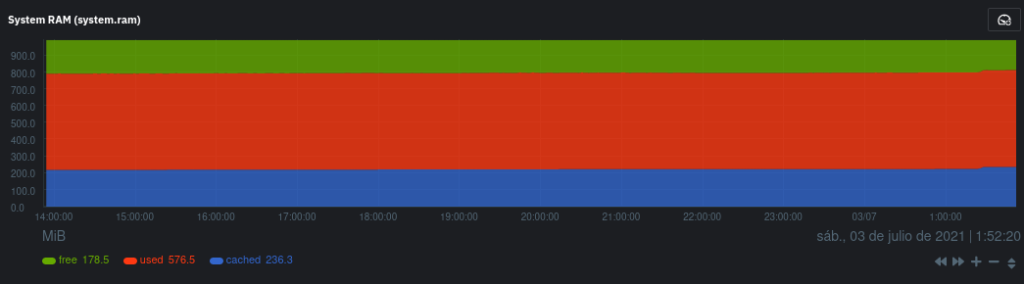
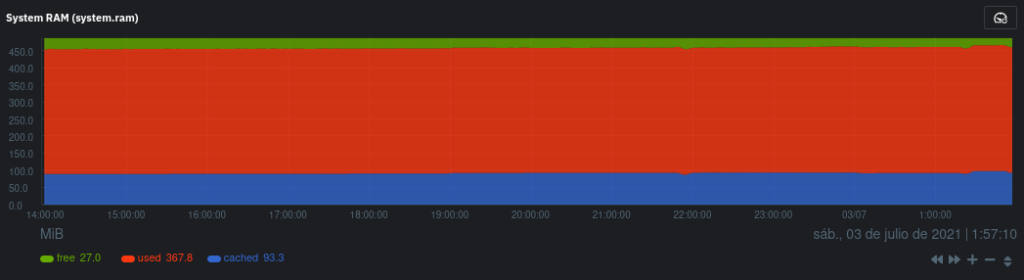
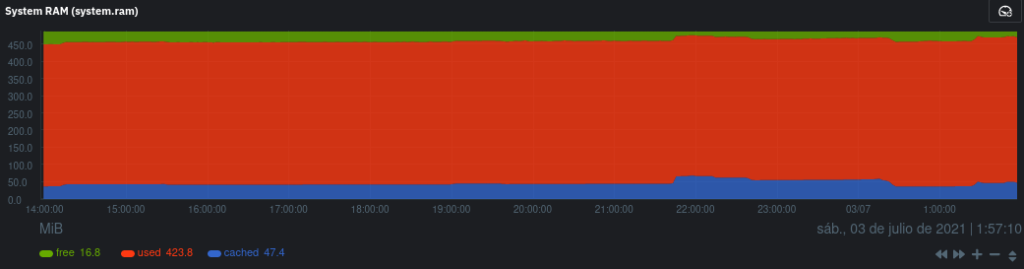
Results
We found that snow2 is the virtual machine that uses the most available hardware resources and is in the second place of bandwidth consumption with 36.73 GiB, after snow1 with 48.84 GiB.
Although there are considerable differences in the hardware configuration between snow 1 and 2, since snow1 has 3G of ram and 2 cores versus 1G and 1 core of snow2, the ratio of CPU consumption stands out. The former is at 20% versus the latter which is at 70%.
Average CPU usage
- snow1 uses on average 20% of CPU
- snow2 uses on average 70% of CPU
- snow3 uses on average 20% of CPU
- snow4 uses on average 20% of CPU
Average ram memory usage
- The ram memory usage of snow1 is 538 MiB, in cache 1.3GiB and free 1.1 GiB
- The ram memory usage of snow2 is 473 MiB, in cache 242MiB and free 276 MiB
- The ram memory usage of snow3 is 364 MiB, in cache 95MiB and free 28MiB
Preliminarily it is identified that the snow 2 hardware configuration is the one that has the best results in relation to its performance and throughput. It delivers the highest amount of bandwidth on the lowest hardware (processing cores and ram memory).
So the snow2 hardware scenario is a good candidate for further analysis in the future, with intermediate scenarios between snow 2 and 1, to identify which hardware features are most ideal in terms of performance and throughput. As well as an understanding and characterization of the snowflake software workload.
The statistical results were shared with the anti-censorship team of the Tor project, the group of people focused on making Tor accessible anywhere in the world, who develop the snowflake software. Within the team, developer meskio highlighted snow2’s ram memory as a relevant aspect of analysis.
References
Hashvis, A Rust application for deterministic generation of images